ACM AI/ML TechTalk Series
Check out the ACM AI/ML TechTalk Series with five distinguished AI/ML researchers.
Anima Anandkumar, Bren Professor of Computing at California Institute of Technology. and Director of Machine Learning research at NVIDIA, explores the challenges of generalizing AI to real-world applications and training data on new, unseen scenarios, domains, and tasks in "How to Create Generalizable AI."
In "The Decision-Making Side of Machine Learning," ACM Fellow and ACM/AAAI Allen Newell Award Winner Michael I. Jordan, Professor of Computer Science and of Statistics at the University of California, Berkeley, focused away from the pattern recognition side of machine learning. Instead, he looked at the statistical, algorithmic, and economic challenges of ML, presenting progress in each area.
Fei-Fei Li, ACM Fellow, Sequoia Capital Professor of Computer Science at Stanford University, and Co-Director of Stanford’s Human-Centered AI Institute, presents the key ideas and cutting edge advances in the quest for visual intelligence in computers, focusing on work done to develop ImageNet over the years in "ImageNet: Where Have We Been? Where Are We Going?"
In "Using Machine Learning to Study Neural Representations of Language Meaning," Tom Mitchell, E. Fredkin University Professor and former Chair of the Machine Learning Department at Carnegie Mellon University (CMU), discusses his machine learning approach to studying how the human brain uses neural activity to create and represent meanings of words, phrases, sentences, and stories.
ACM Fellow Eric Horvitz, Technical Fellow and Director of Microsoft Research Labs, shares reflections on promising directions with fielding AI solutions in the open world, where systems need to grapple with uncertainty and incompleteness and to work effectively with people in "AI, People, and the Open World."
Lifelong Learning
ACM offers lifelong learning resources including online books and courses from Skillsoft, TechTalks on the hottest topics in computing and IT, and more.
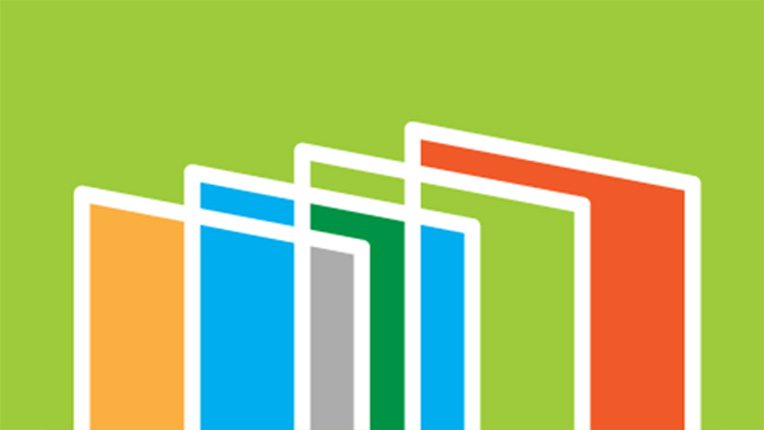